Overview
This course builds upon and extends the DevOps practice prevalent in software development to build, train, and deploy machine learning (ML) models. The course stresses the importance of data, model, and code to successful ML deployments. It will demonstrate the use of tools, automation, processes, and teamwork in addressing the challenges associated with handoffs between data engineers, data scientists, software developers, and operations. The course will also discuss the use of tools and processes to monitor and take action when the model prediction in production starts to drift from agreed-upon key performance indicators. The instructor will encourage the participants in this course to build an MLOps action plan for their organization through daily reflection of lesson and lab content, and through conversations with peers and instructors
Who should take this course
- ML data platform engineers
- DevOps engineers
- Developers/operations staff with responsibility for operationalizing ML models
Prerequisites
Required:
- AWS Technical Essentials course (classroom or digital)
- DevOps Engineering on AWS course, or equivalent experience
- Practical Data Science with Amazon SageMaker course, or equivalent experience
Recommended:
- The Elements of Data Science (digital course), or equivalent experience
- Machine Learning Terminology and Process (digital course)
Delegates will learn how to
- Describe machine learning operations
- Understand the key differences between DevOps and MLOps
- Describe the machine learning workflow
- Discuss the importance of communications in MLOps
- Explain end-to-end options for automation of ML workflows
- List key Amazon SageMaker features for MLOps automation
- Build an automated ML process that builds, trains, tests, and deploys models
- Build an automated ML process that retrains the model based on change(s) to the model code
- Identify elements and important steps in the deployment process
- Describe items that might be included in a model package, and their use in training or inference
- Recognize Amazon SageMaker options for selecting models for deployment, including support for ML frameworks and built-in algorithms or bring-your-own-models
- Differentiate scaling in machine learning from scaling in other applications
- Determine when to use different approaches to inference
- Discuss deployment strategies, benefits, challenges, and typical use cases
- Describe the challenges when deploying machine learning to edge devices
- Recognize important Amazon SageMaker features that are relevant to deployment and inference
- Describe why monitoring is important
- Detect data drifts in the underlying input data
- Demonstrate how to monitor ML models for bias
- Explain how to monitor model resource consumption and latency
- Discuss how to integrate human-in-the-loop reviews of model results in production
Outline
Day 1
Module 0: Welcome
- Course introduction
Module 1: Introduction to MLOps
- Machine learning operations
- Goals of MLOps
- Communication
- From DevOps to MLOps
- ML workflow
- Scope
- MLOps view of ML workflow
- MLOps cases
Module 2: MLOps Development
- Intro to build, train, and evaluate machine learning models
- MLOps security
- Automating
- Apache Airflow
- Kubernetes integration for MLOps
- Amazon SageMaker for MLOps
- Lab: Bring your own algorithm to an MLOps pipeline
- Demonstration: Amazon SageMaker
- Intro to build, train, and evaluate machine learning models
- Lab: Code and serve your ML model with AWS CodeBuild
- Activity: MLOps Action Plan Workbook
Day 2
Module 3: MLOps Deployment
- Introduction to deployment operations
- Model packaging
- Inference
- Lab: Deploy your model to production
- SageMaker production variants
- Deployment strategies
- Deploying to the edge
- Lab: Conduct A/B testing
- Activity: MLOps Action Plan Workbook
Day 3
Module 4: Model Monitoring and Operations
- Lab: Troubleshoot your pipeline
- The importance of monitoring
- Monitoring by design
- Lab: Monitor your ML model
- Human-in-the-loop
- Amazon SageMaker Model Monitor
- Demonstration: Amazon SageMaker Pipelines, Model Monitor, model registry, and Feature Store
- Solving the Problem(s)
- Activity: MLOps Action Plan Workbook
Module 5: Wrap-up
- Course review
- Activity: MLOps Action Plan Workbook
- Wrap-up
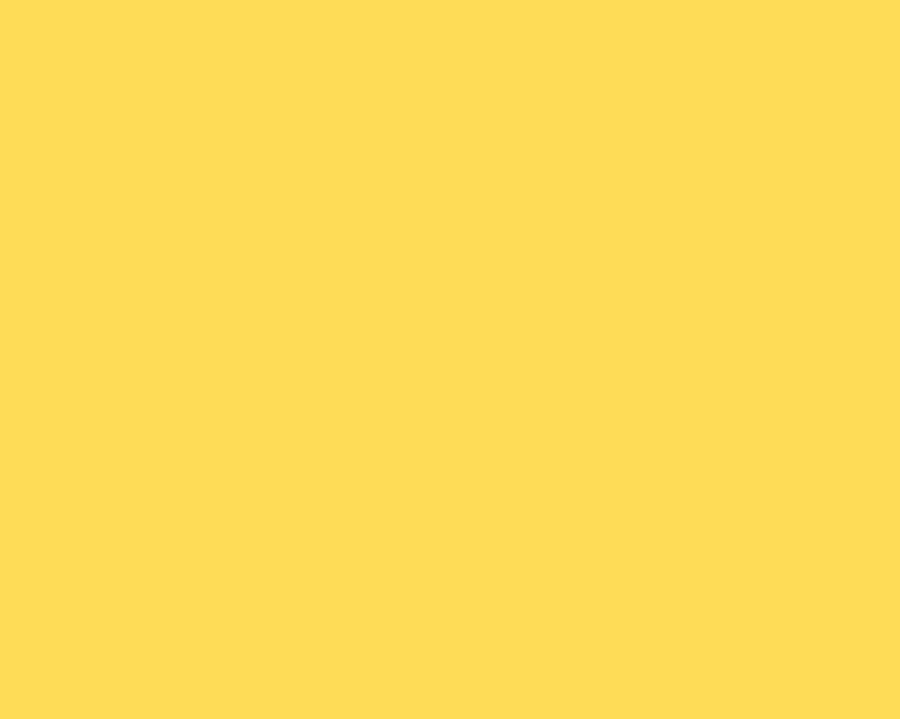
Frequently asked questions
How can I create an account on myQA.com?
There are a number of ways to create an account. If you are a self-funder, simply select the "Create account" option on the login page.
If you have been booked onto a course by your company, you will receive a confirmation email. From this email, select "Sign into myQA" and you will be taken to the "Create account" page. Complete all of the details and select "Create account".
If you have the booking number you can also go here and select the "I have a booking number" option. Enter the booking reference and your surname. If the details match, you will be taken to the "Create account" page from where you can enter your details and confirm your account.
Find more answers to frequently asked questions in our FAQs: Bookings & Cancellations page.
How do QA’s virtual classroom courses work?
Our virtual classroom courses allow you to access award-winning classroom training, without leaving your home or office. Our learning professionals are specially trained on how to interact with remote attendees and our remote labs ensure all participants can take part in hands-on exercises wherever they are.
We use the WebEx video conferencing platform by Cisco. Before you book, check that you meet the WebEx system requirements and run a test meeting (more details in the link below) to ensure the software is compatible with your firewall settings. If it doesn’t work, try adjusting your settings or contact your IT department about permitting the website.
How do QA’s online courses work?
QA online courses, also commonly known as distance learning courses or elearning courses, take the form of interactive software designed for individual learning, but you will also have access to full support from our subject-matter experts for the duration of your course. When you book a QA online learning course you will receive immediate access to it through our e-learning platform and you can start to learn straight away, from any compatible device. Access to the online learning platform is valid for one year from the booking date.
All courses are built around case studies and presented in an engaging format, which includes storytelling elements, video, audio and humour. Every case study is supported by sample documents and a collection of Knowledge Nuggets that provide more in-depth detail on the wider processes.
When will I receive my joining instructions?
Joining instructions for QA courses are sent two weeks prior to the course start date, or immediately if the booking is confirmed within this timeframe. For course bookings made via QA but delivered by a third-party supplier, joining instructions are sent to attendees prior to the training course, but timescales vary depending on each supplier’s terms. Read more FAQs.
When will I receive my certificate?
Certificates of Achievement are issued at the end the course, either as a hard copy or via email. Read more here.